Table of Contents:
Stand up straight. Close your eyes. Rock back on your heels and get ready to fall. Don’t worry – your colleagues will be there to catch you.
Won’t they?
Executive Summary
Artificial Intelligence (AI) can do incredible things for insurance companies. But unless you’re approaching it in the right way, using AI can feel a bit like taking a trust fall.
It sounds safe enough on paper, but what happens if something goes wrong? Will the reassurances you’ve been given stand up to scrutiny? Or will you be the one who hits the ground with a bump?
Today, it isn’t just data scientists and AI researchers that need to worry about the responsible use of AI. As regulation becomes increasingly stringent, everyone at every level of the insurance industry needs to know that the models they’re using adhere to the rules.
In this guide, we’ll look at the biggest questions that insurers face about their use of artificial intelligence, and why now is the time for them to embrace the concept of AI governance.
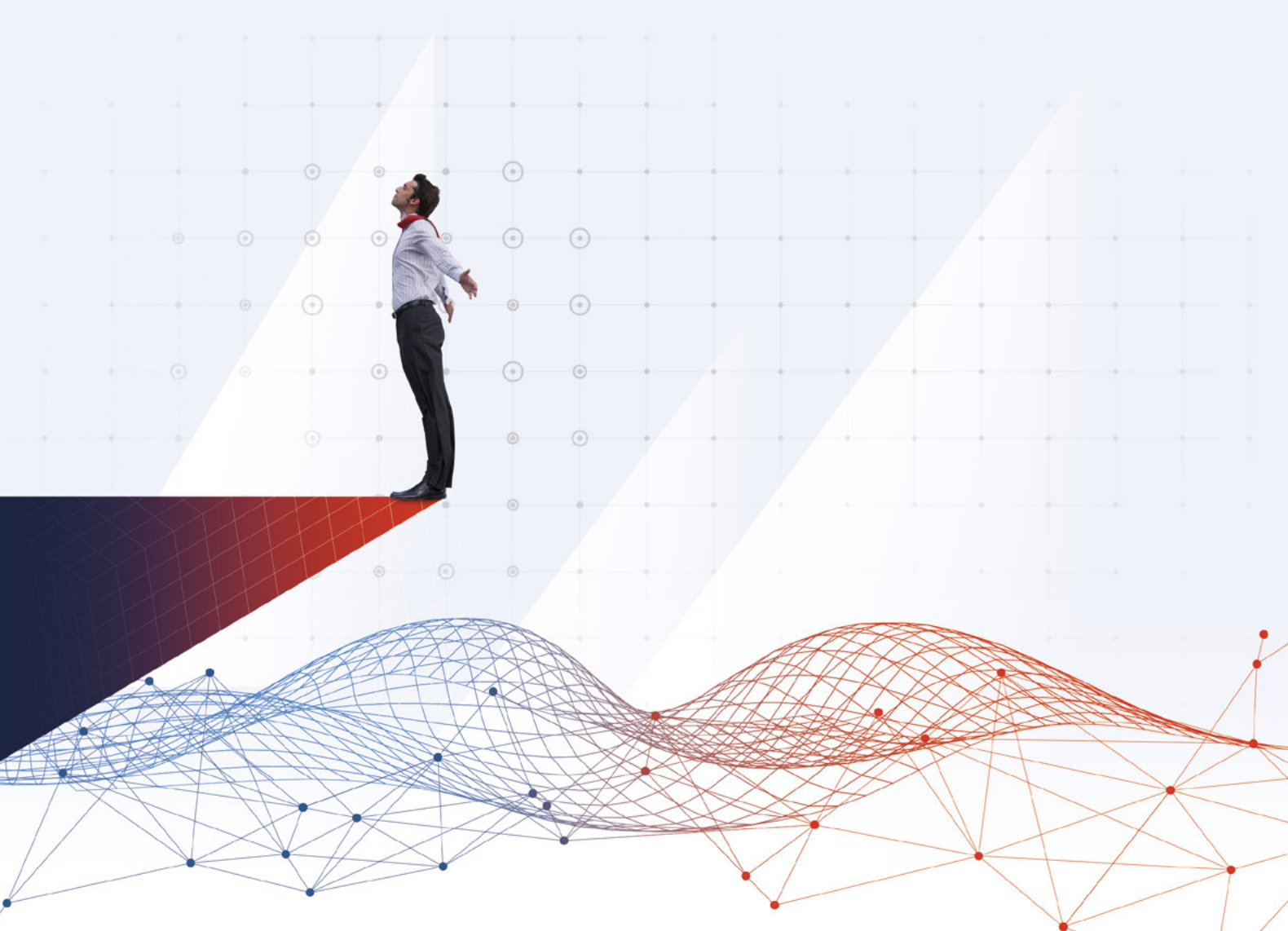
Introduction
Around the world, insurers are going all in on AI. From risk assessment to claims processing, fraud detection to product innovation, insurance companies are using AI to enhance their operations, improve customer service, and get a step up on the competition.
Pricing is no different. Not only are AI models extremely capable when it comes to processing large volumes of data, they are also excellent at extracting information and detecting patterns. Because of this, AI is helping insurance companies develop more agile and accurate pricing models, even in the face of an extremely dynamic market environment. This ability to analyse vast data sets is also crucial in the fight against fraud with AI models employed to identify unusual patterns and anomalies as part of wider prevention strategies.
While many of the AI-powered applications in use at insurance companies have been licensed from third parties, pricing models are typically developed in-house. Partly as a result of the data involved, and partly because doing so empowers genuine competitive advantage.
As the technology matures and AI adoption grows, things are changing. The costs of managing AI models is increasing and the gap’s growing between the small number of insurers with the technical skills and resources to manage AI at scale and everyone else. This means that many are struggling to compete.
Creating (and managing) an AI model is about more than just big teams and technical capability, though. It’s about making sure that the model is responsible, ethical, and aligned with business strategies and current regulations, too. And the more models you have, the harder these tasks become.
For insurance leaders a few steps removed from the technology, it can be difficult to know whether those requirements are being met. Without direct insight into how a model has been created, the only thing you have to go on are promises and reassurances. As any regulatory will tell you, those aren’t always enough.
So, what does that mean in terms of risk and exposure? And what questions should leaders ask to ensure that your own AI models don’t cause trouble down the line?
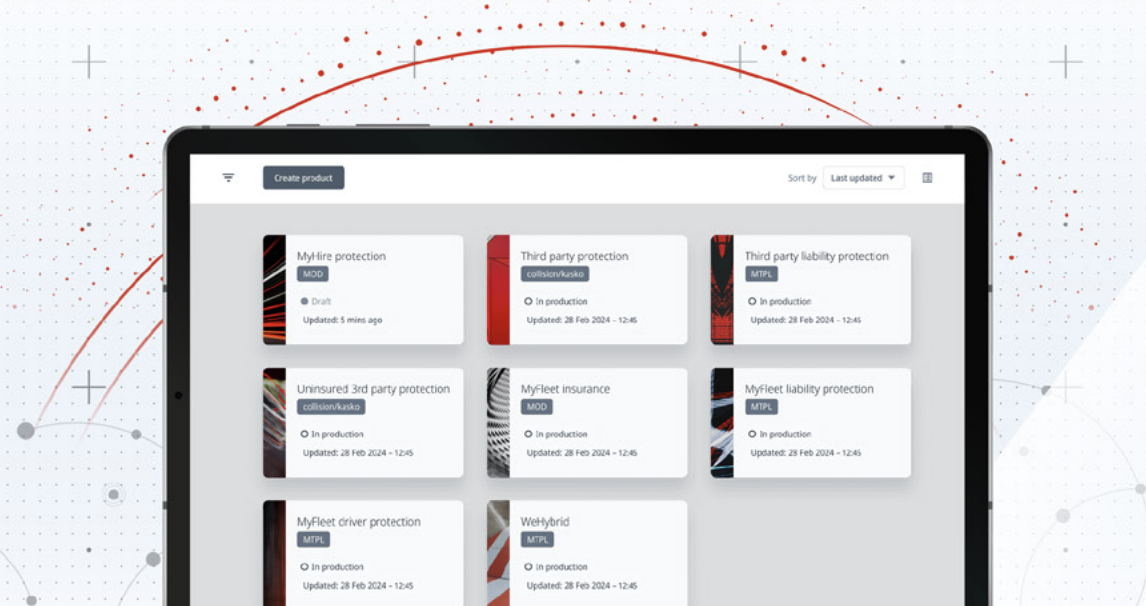
Regulation of AI in the UK: What you need to know
AI Regulation in the UK is moving quickly. As of today:
- The UK government published its AI Regulation White Paper in March 2023, outlining a proposed regulatory framework.
- At the core of that framework are five key principles, including transparency, explainability, and fairness.
- Many of the UK’s regulators—the Financial Conduct Authority (FCA) included—are drawing up sector-specific guidelines around the use of AI.
- The FCA’s recent “AI Update” specifies that “the Senior Managers and Certification Regime (SM&CR)” emphasises senior management accountability and is relevant to the safe and responsible use of AI.
- Further, the FCA states that “any use of AI in relation to an activity, business area, or management function of a firm would fall within the scope of a Senior Management Function manager’s responsibilities.”

Concerning Bias
Bias is hardly a new challenge for the insurance industry. 30 years ago, one of North America’s largest insurance companies was forced to respond to claims that it was ‘denying policies to African American and Hispanic homeowners and residents of older inner-city neighbourhoods’. In the years since, insurers around the world have been dogged by similar claims of discrimination - deliberate or otherwise.
While bias might be an age-old issue - Citizens Advice found people of colour pay £250 more for car insurance in the UK - the proliferation of AI has rightly kept the issue in the spotlight.
AI has its own well-documented problems with this issue. There’s the fact that AI image generators tend to associate higher-income roles like a lawyer or CEO with lighter skin tones, for instance. In the U.S. for example, notable class actions suits have been brought against insurers—with one lawsuit claiming State Farm’s algorithms were biased against African American names.
All of this begs one obvious question: how do you know that your own AI model isn’t making decisions that are to the detriment of some of your customers?
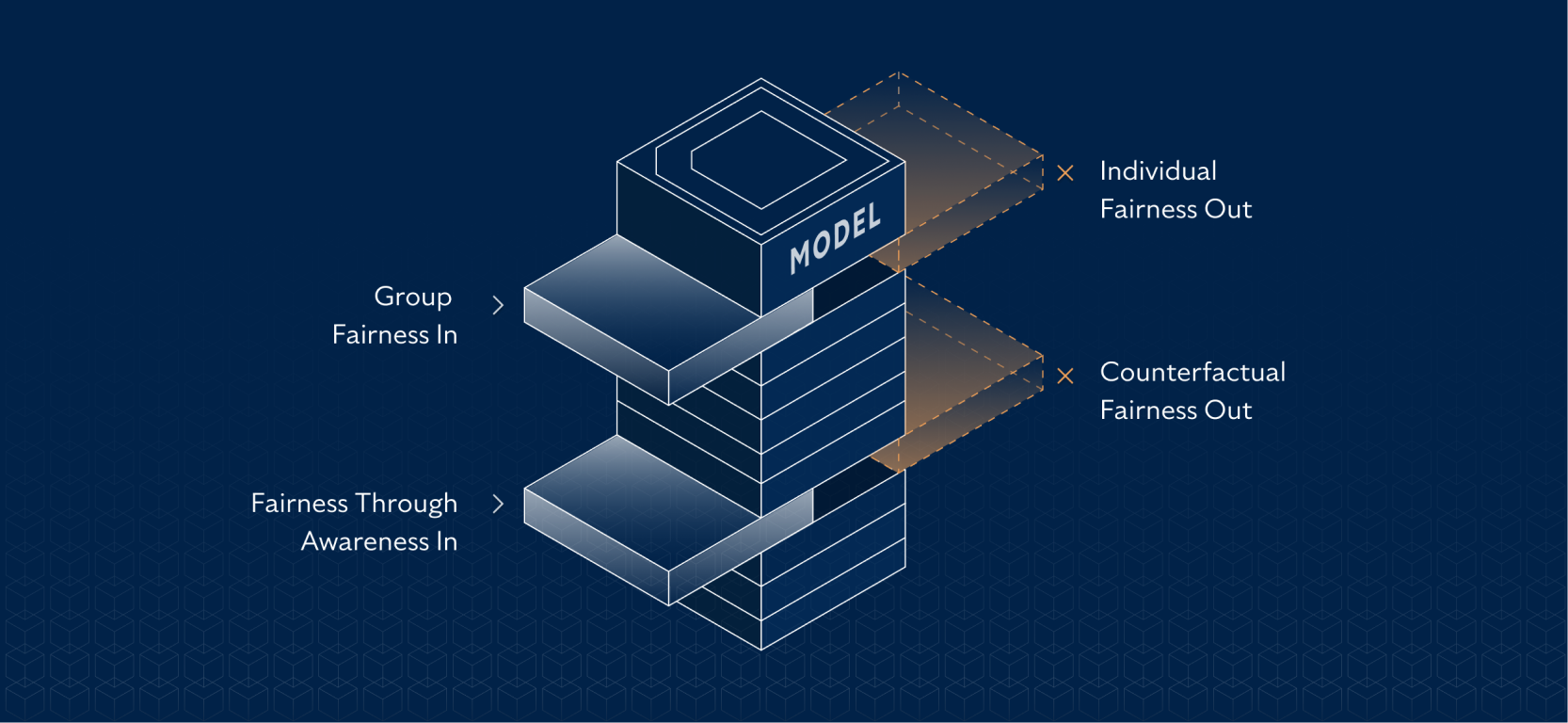
Question 1: What have we done to minimise bias in our AI model?
To minimise unwanted bias, you first need to be able to detect it - and that can be easier said than done.
An algorithm doesn’t become biased by itself. Bias can be caused by anything from the data that it’s trained on through to assumptions made by the people developing it. What’s more, if a biased model is then used to train subsequent iterations, those inherent prejudices will be perpetuated and amplified. So, understanding where a particular bias originates is a challenge in itself.
The other consideration here is that there are a range of different ways to define fairness of treatment:
- Group fairness: parity between different protected groups such as gender or race.
- Individual fairness: with similar individuals being treated in a similar way.
- Awareness-based fairness: where individuals who are similar in respect to a particular task should be classified similarly.
- Counterfactual fairness: where an individual would receive the same treatment in the real world and a “counterfactual” world in which they belonged to a different demographic group.
Even then, it can be difficult to ensure fairness across all of those categories. Individual fairness can clash with the principles of group fairness, for instance. Nonetheless, knowing that these issues have been taken into account should provide some reassurance that bias has been reduced - if not wholly eliminated.
Transparent and explainable AI models allow insurers to identify discrimination and bias at source and take preventative action before they harm their customers and, through regulatory intervention and sanctions, their business.
Put it another way: bias isn’t always a bad thing. Pricing only works because it is biased - based on the individual’s attributes. The key lies in making sure that this bias is applied fairly and legally.
With the right approach to model transparency and explainability and some AI-assisted techniques that make complex models more explainable, insurers can identify potentially dangerous issues like discrimination and unfair bias within individual models, and can continue to do so as they scale their AI use.
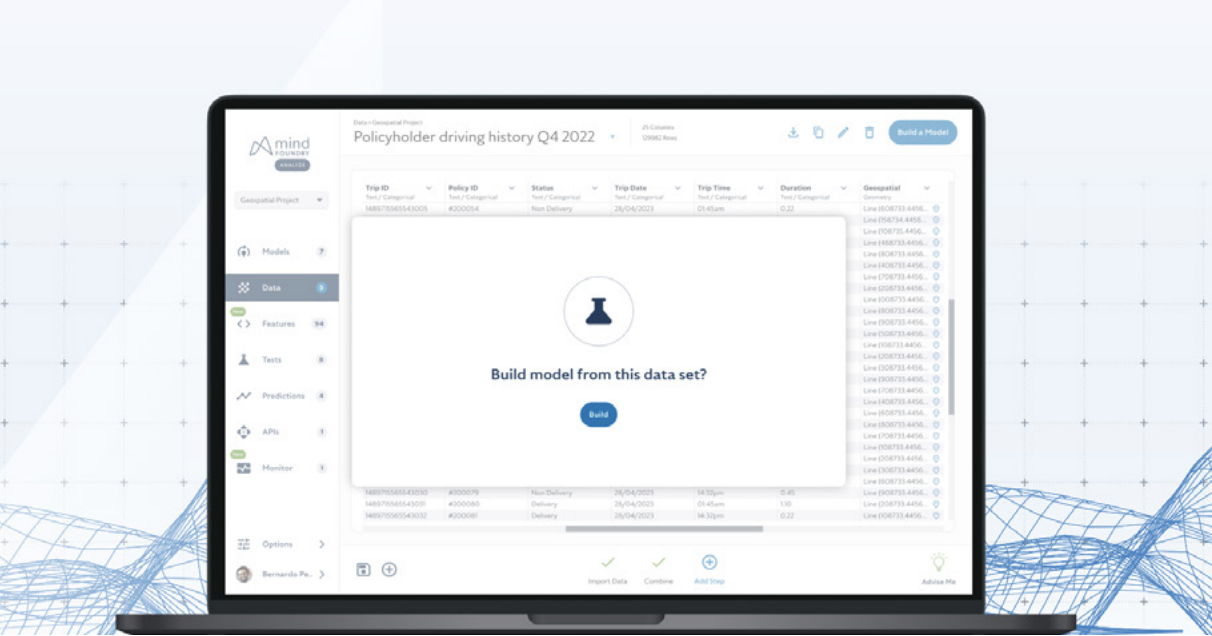
Uncovering Explainability
In 2021, 19 insurance companies signed up to the Explaining Underwriting Decisions (EUD) agreement. Created by the Access to Insurance Group, the EUD is designed to provide insurance customers with greater insight into the underwriting decision-making process. Specifically, it seeks to help those with pre-existing conditions understand why they’ve been declined, postponed, or rated in a certain way.
Clearly, the EUD represents a major step forward for the industry. Moreover, it speaks to the growing importance of explainability within insurance as a whole.
Explainability is similarly important when it comes to AI. One of the major criticisms levelled at AI models is that they act as a “black box” - one in which even the people who developed them have little idea why decisions have been made in a certain way. Data goes in, and a recommendation comes out, but with little insight into how it was reached. That’s hardly reflective of the kind of transparency that many insurers are now aiming to provide.
This leads one inevitable question if you’re using AI within your own business: could you explain to your customers and regulators how your AI model makes its decisions?
Explainability has a other business benefits as well. The ability to see and understand the decision-making data in the model may provide insurers with new insights to inform the development of more personalised series or new product offers. Indeed, models can also be combined to solve new problems and learnings distributed across the portfolio—not only maintaining performance levels but improving over time to increase value generation and sharing the benefits of AI across the business.
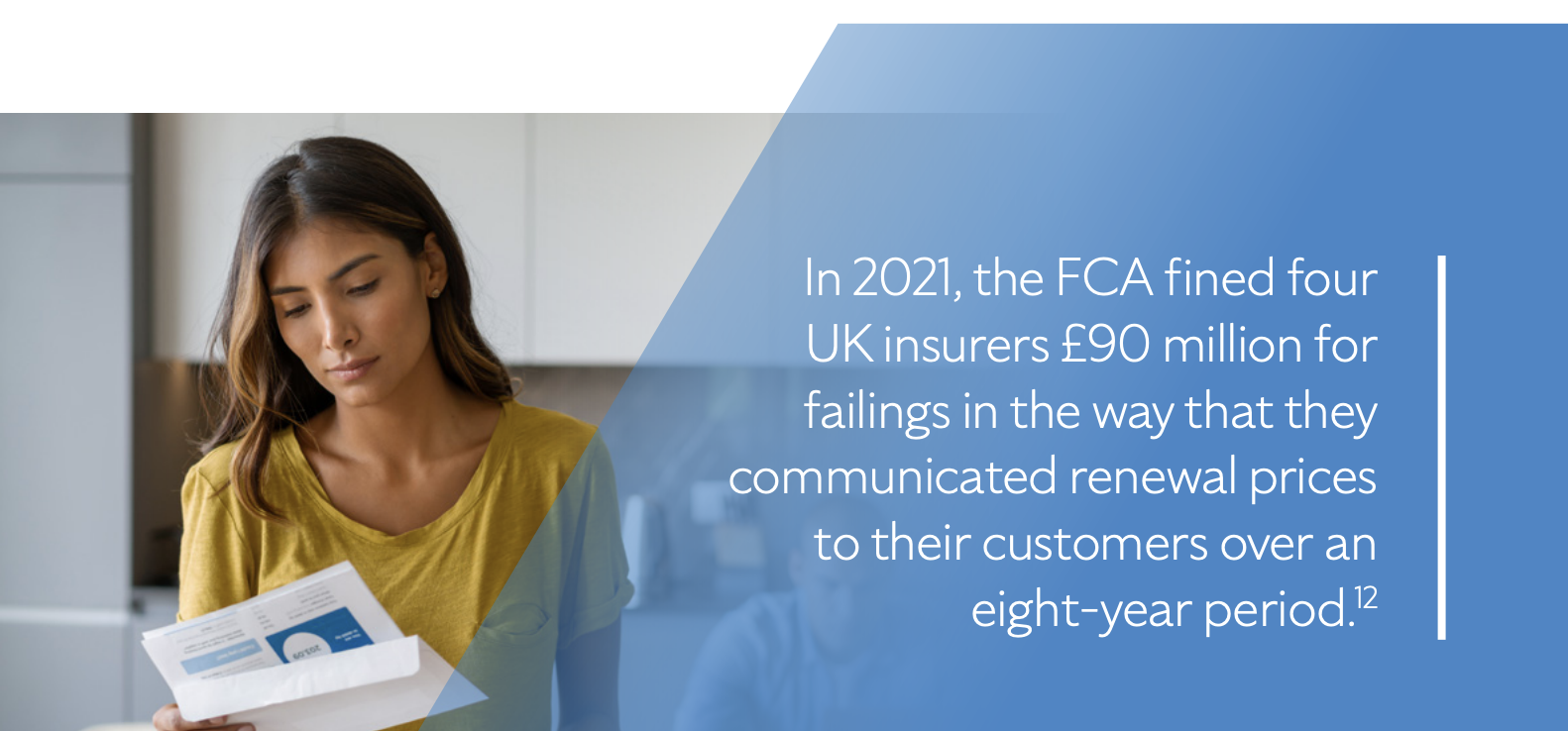
Question 2: Can we explain how our AI model works?
The subject of explainable AI (or XAI) has grown in importance and urgency with the arrival of the Consumer Duty, a new set of rules and guidance overseen by the FCA. Amongst other things, the Consumer Duty specifies that consumers must “receive communications they can understand [and] products and services that meet their needs and offer fair value.”
In practice, that means one thing: insurers will be under growing pressure to state, in very clear terms, why a certain decision was made - regardless of whether it was a machine or a person that made it.
One of the key challenges here is finding the right balance between sophistication and transparency, particularly around pricing. Traditional pricing models (generalised linear models, for instance) offered at least some degree of visibility. For pricing insurance, for example, a GLM can incorporate easily interpretable (and explainable) data such as driver age, driving experience, past claims history and location.
In the same pricing scenario, more sophisticated models (like gradient-boosted models) tend to be far more opaque—incorporating a range of additional factors including, but not limited to, real-time driving behaviour, weather patterns, traffic levels and so on. This is certainly the direction of travel within insurance as GBMs help providers insurers carve out an edge over the competition. However, it’s also true that many find it hard to explain how each factor is weighted. They’re not called ‘black boxes’ for nothing.
Of course, there are different levels of explainability to consider here too. While a technical specialist might want to understand the deep intricacies of a predictive model, an end customer almost certainly won’t. Instead, they’ll want an easy-to-understand explanation of why their premiums are priced in a certain way.
Ultimately, what really matters is that the people who build, manage, or use your AI model understand how it works—and can communicate the decisions it makes in a clear and simple way. After all, understanding how or why a model works is not just for the data scientists.
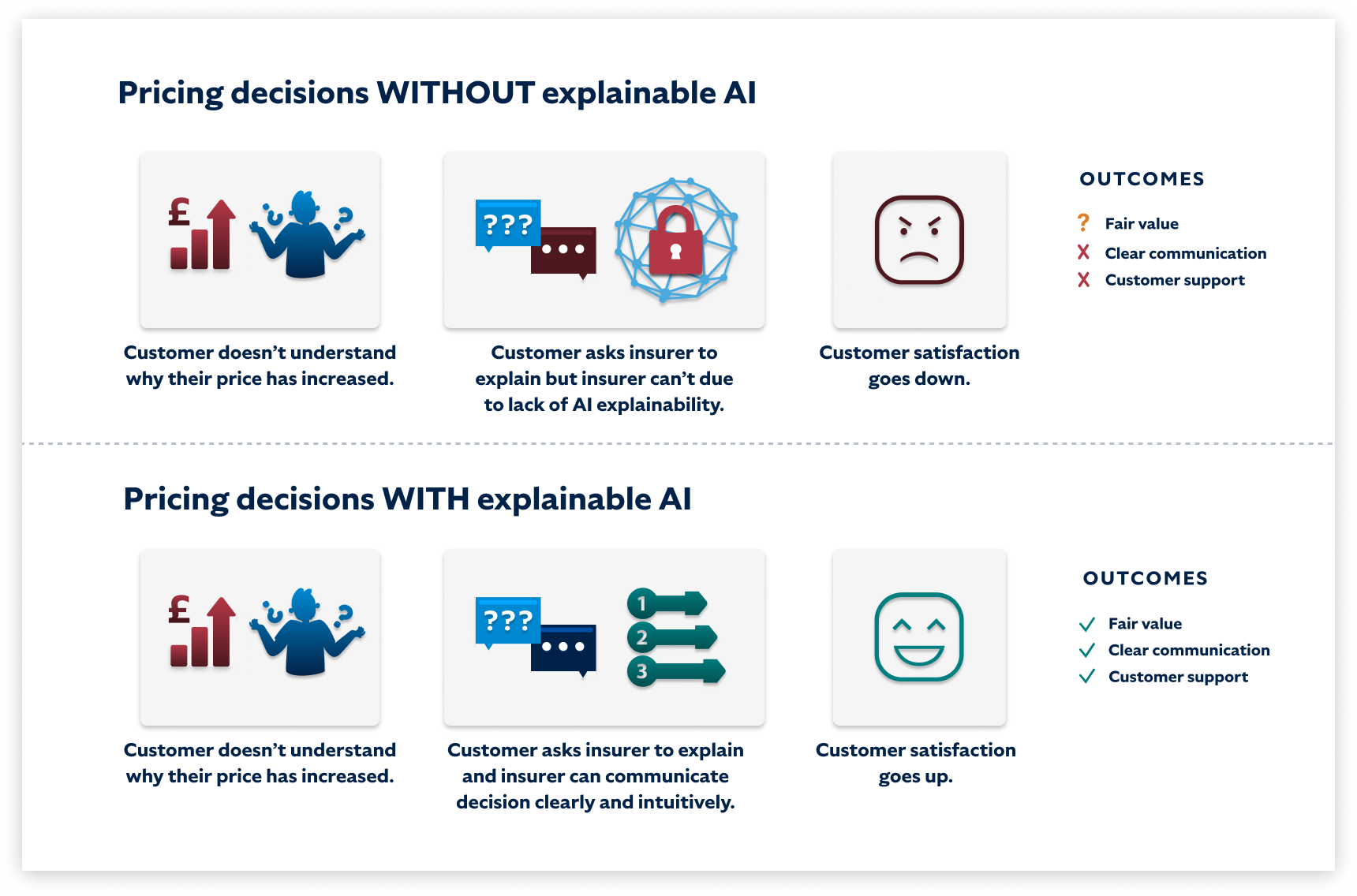
Managing Scale
So far, we’ve talked about AI in the singular—as if insurers only have one AI model to worry about.
The reality, of course, is usually very different. While some providers (early in their AI journeys) may be able to rely on just one or two models, most insurers will need dozens, if not hundreds, of models to remain competitive. In highly competitive and rapidly changing use cases like pricing, a mature insurer could be running many hundreds of different models just for this alone.
Naturally, managing AI at that scale is challenging. More models can make for a greater overall opportunity, but they inevitably lead to greater complexity, too. That’s true not just in terms of the issues outlined above - with insurers needing to think about things like bias and explainability across multiple models - but in terms of upkeep and maintenance, too.
Insurance is a dynamic industry. From new risks and changing consumer behaviours, through to regulatory shifts and evolving market conditions, insurers are deluged with new information daily. To ensure that their AI systems continue to perform as things around them change, insurers need to feed that information in - a problem in itself considering that traditional models need hundreds, if not thousands of labelled examples to “understand” a new pattern or class.
Insurers must set clear operational bounds and KPIs to validate model performance and understand how AI impacts their business. They should also benchmark for reliability and consistency and implement feedback loops to facilitate continuous improvement. Individual model monitoring protocols like drift detection should also be considered a fundamental component of AI adoption, as without them, it can be impossible to identify model performance decline until it starts negatively impacting the bottom line. Insurance leaders should also be mindful that achieving all this is incredibly difficult when attempted entirely in-house. There are external solutions that can facilitate proper AI governance, and insurance leaders should consider all the tools at their disposal to make their AI adoption successful.
It’s with that thought in mind that anyone putting their trust in AI should be asking: “what are we doing to keep this model up to date?”
There’s also a people element at play here. Data science teams must spend more time and effort retraining and maintaining existing models - rather than helping insurers scale their AI with new ones. These are repetitive tasks that highly qualified individuals do not like doing. Which perhaps goes some way to explaining why the average data scientist will leave their current role after just 1.7 years.
Retaining data science talent is a huge challenge for insurers. Finding alternative (and automated) approaches to fully in-house model building and management will go a long way to solving both scale and retention challenges.
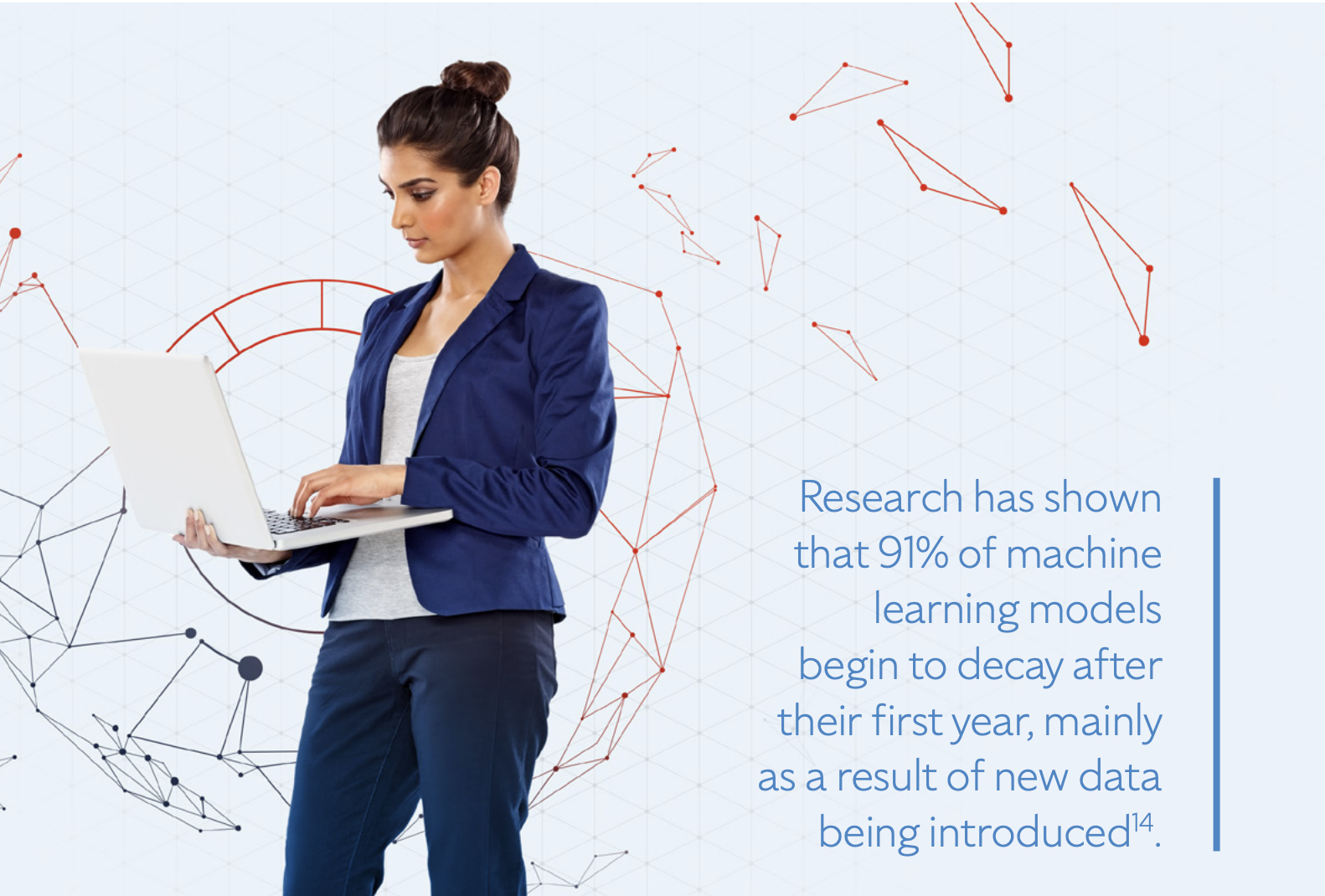
Question 3: How are we keeping our AI model up to date?
Imagine you’ve just hired a new employee. Now think about your expectations for their performance over time. Naturally, it’ll take them a little time to get up to speed with their new environment. There will be parts of the job that they’ll only learn through experience, too. Within a few weeks or months, though, they’d normally be well on top of things and doing their job to the best of their abilities.
AI models work in an almost completely opposite fashion. Typically, their first “day on the job” is also their best, with new data and changing external circumstances gradually causing a model to become less and less effective. This is a problem that’s typically referred to as “drift”.
Drift can be tackled, but not easily. To bring it up to speed again, a model will typically need to be retrained or rebuilt—both of which can be costly activities. It’s because of this that AI governance capabilities and continuous model management have become so important.
Retraining or replacing a model when it inevitably starts to experience performance decline is expensive, and the unpredictable nature and timing of this expense poses a headache for budget holders. Implementing the necessary model explainability, transparency, and measures for continuous model monitoring can also enable data science teams to focus on more important tasks. It also means that problems like data drift are identified and rectified before they can negatively impact the bottom line.
AI governance: the time is now
Responsible. Ethical. Compliant. As insurers operationalise AI at scale, they also need to make sure that their models play by the rules - and can be proven to do so.
Increasingly, the smart way to do this is through AI governance. A set of principles or processes designed to ensure that AI is developed and deployed in an appropriate way, AI governance provides a way to assess the potential impact on end-users, customers, and the enterprise as a whole. Some resources to consider include the Association of British Insurers (ABI) guide to getting started with responsible AI and our piece on 5 Ways to Manage AI Responsibly in Insurance.
As with any other kind of governance, this is a multidisciplinary concern. To be truly effective, AI governance requires multiple stakeholders from across your business to come together and ensure that the system or model in question meets their needs. Whether it’s data scientists, actuaries, pricing managers, compliance officers, or others, anyone who has a link to your AI model must provide their views.
Effective AI governance requires effort - but that effort will be increasingly worthwhile. As much as AI may be under the microscope today, tomorrow will only bring greater attention.
As that happens, a proactive approach to AI governance will ensure that you can continue to operationalise AI in a safe, responsible, and sensible way.
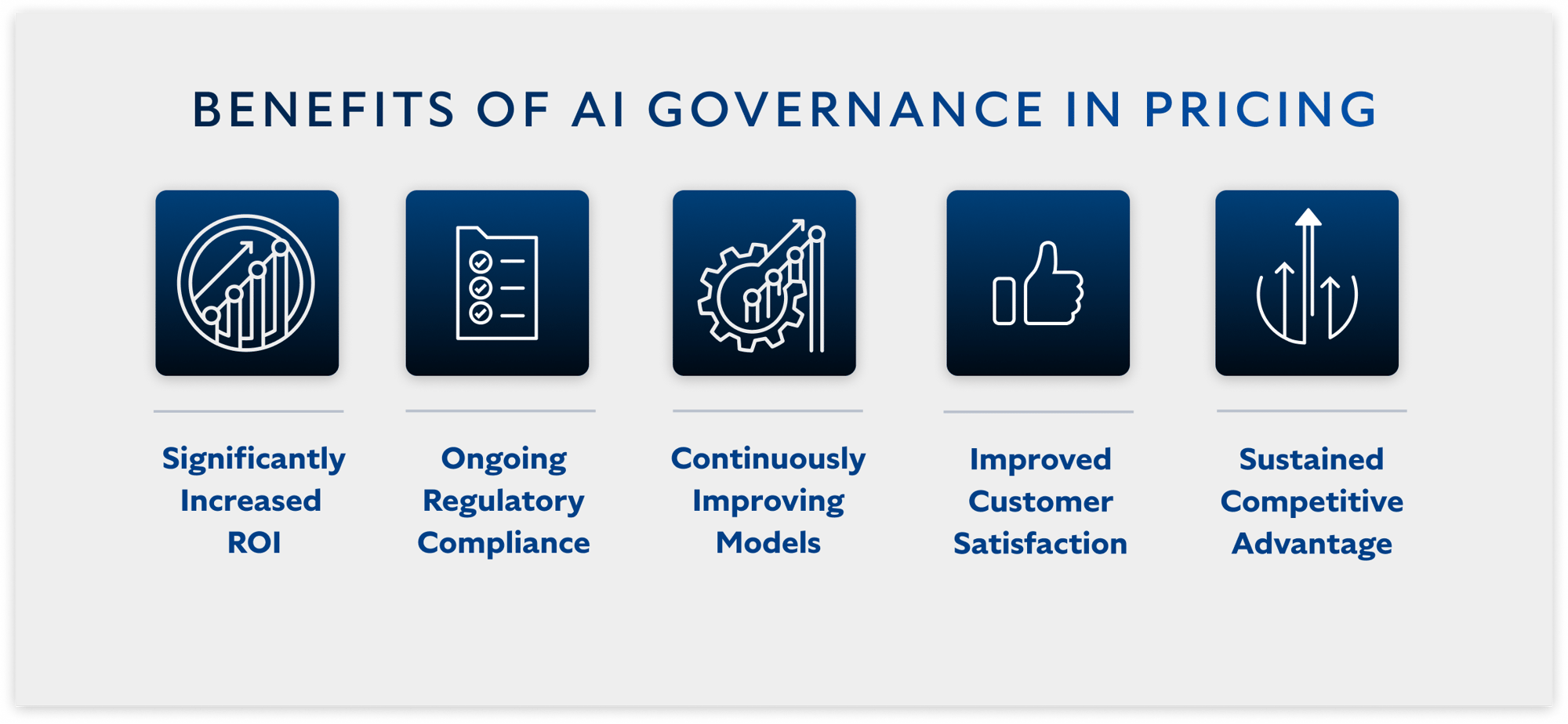
Get the answers you need
As a ferociously competitive industry, insurers can’t afford to fall behind the times and allow their competitors to beat them to the benefits of innovation.
The right strategies and tools for AI adoption in insurance can deliver the competitive advantage insurers require, with models that maximise profit margins, maintain regulatory compliance, deliver sufficient explainability to keep customers happy, and ultimately provide the foundation to grow their AI portfolio.
The regulatory response to the risks posed by AI has placed significant responsibility on insurance leaders. Just as AI can help maximise profits and reduce losses, it can compromise both those goals when adopted without the right considerations. AI governance is a key part of the solution to this problem.
The responsibility for AI strategy falls on key decision-makers who must navigate regulatory requirements, manage risks, build customer trust, protect data privacy, improve operational efficiency, and gain a competitive advantage. With the right strategies for its adoption, AI’s risks can be effectively mitigated whilst its benefits can be maximised. Insurance leaders must implement these strategies to be at the forefront of the AI insurance revolution.
Contact us today
Learn more about how we can help you bring the benefits of AI to your organisation.
4 min read
AI Insights from Insurance Leaders in 2024
by Mind Foundry
8 min read
AI vs Fraud in the UK and Japan
by Nick Sherman
Stay connected
News, announcements, and blogs about AI in high-stakes applications.