Case Study
AI in Construction
Using AI to reduce injury and save lives on construction sites.
Problem:
BAM Nuttall, a large construction and civil engineering company, had access to data on historic incidents and the conditions and situations surrounding them but struggled to extract actionable insight from this information. In collaboration with the Health and Safety Executive (HSE), they wanted to discover the key causal factors of accidents and use this knowledge to take preemptive action on future projects and reduce the risk of injury to their workforce.
Solution:
To extract actionable insight from their datasets, BAM Nuttall chose the Mind Foundry Platform; an end-to-end Machine Learning platform that synthesises cutting-edge AI and human expertise to build real-world solutions for unique problems.
The Platform empowered users to build powerful predictive models and explore hidden insights within their data in a friendly, no-code environment. This enabled health and safety experts to interact with an AI and Machine Learning solution in a way that amplified their expertise.
Mind Foundry was able to surface a number of causal relationships between incident severity, type, and environmental conditions. This analysis helped quantify the safety impact of undertaking work at different times of year with different partners, as well as other factors. Understanding these relationships allowed the client to be proactive in monitoring at-risk individuals and projects more thoroughly to maximise care and minimise risk.
Combining this data with historic health and safety inspection scores resulted in models that reliably predicted the outcome of health and safety inspections before they took place.
Results:
Mind Foundry’s solution enabled users to explore the data in ways that would have otherwise been impossible due to the nature and size of the datasets. This included determining whether project characteristics, such as the growing number of people on-site, affected safety over time or if these features were linked with other observed health and safety risks.
Accurate predictions from the resultant model allowed them to monitor current projects and send inspection teams to those most at risk of scoring poorly on an inspection, thus catching and remedying potential problems before they caused injury.
A primary challenge was to spot accident patterns using a variety of data sources. Of the 17 types of injuries analysed, Mind Foundry found that "time of year," "level of experience," and "age" were the leading influencers of most injuries.
Understanding the main determining factors behind accidents enabled the client to quantify the safety impact of undertaking work at different times of year with different partners and to be proactive in monitoring at-risk individuals and projects more thoroughly to maximise care and minimise risk.
Download the full case study
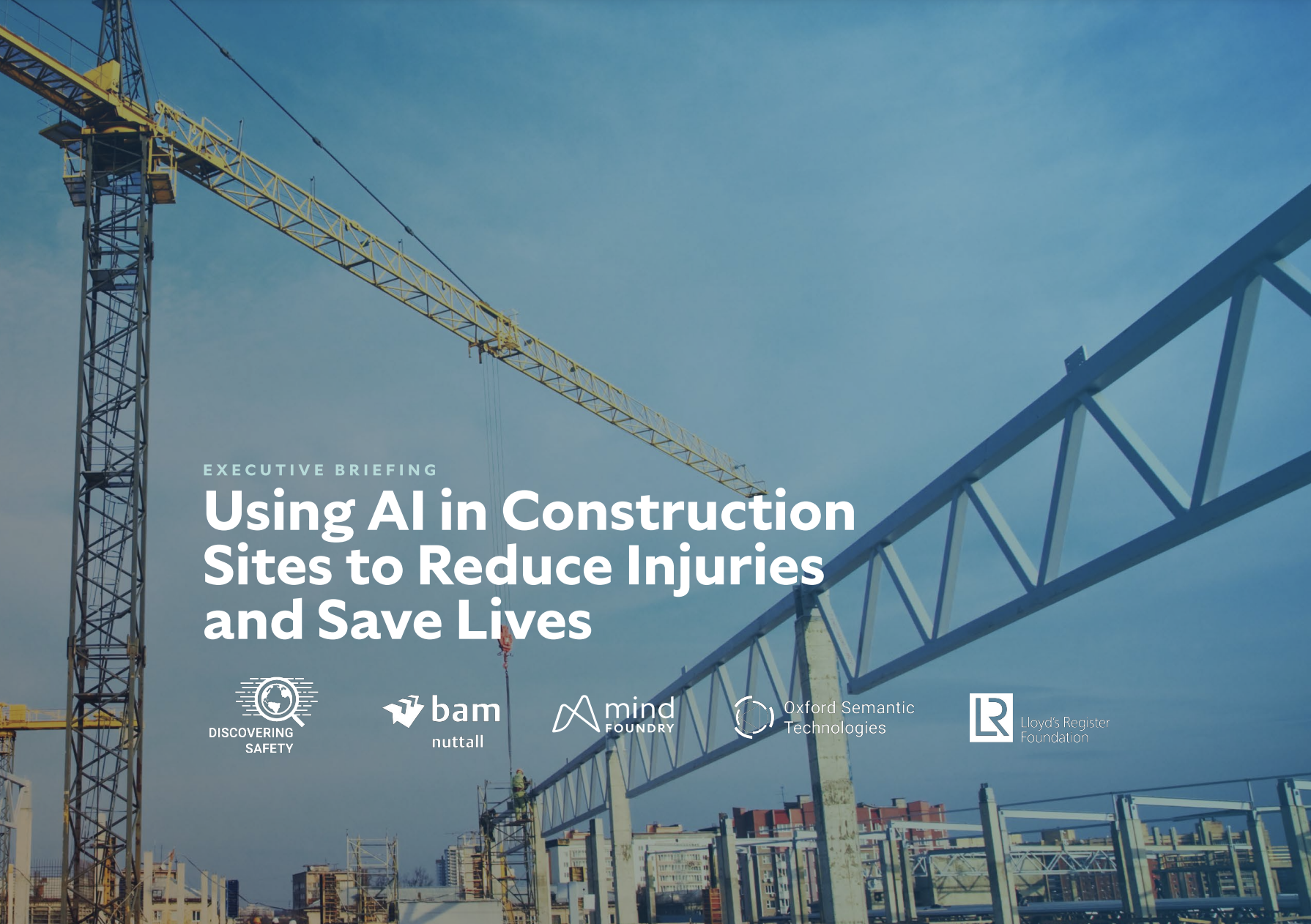
.png?width=2000&name=BamNuttal%20(1).png)
“Combining data sets without direct relationships is possible and has the potential to generate useful insights and help to identify previously unrecognised relationships.”
Phil Cullen
Director of Health & Safety
Contact us for more information
6 min read
The Business of AI in UK Defence and National Security
by Al Bowman
4 min read
The 8-Step Guide to Deploying Machine Learning in Infrastructure
by Kimberly Joly
Stay connected
News, announcements, and blogs about AI in high-stakes applications.