Mind Foundry Products
Responsible AI products that enhance decision-making, strengthen frontline operations and safeguard critical infrastructure.
Designed for deployment.
Enabling real-time trusted intelligence in the toughest environments
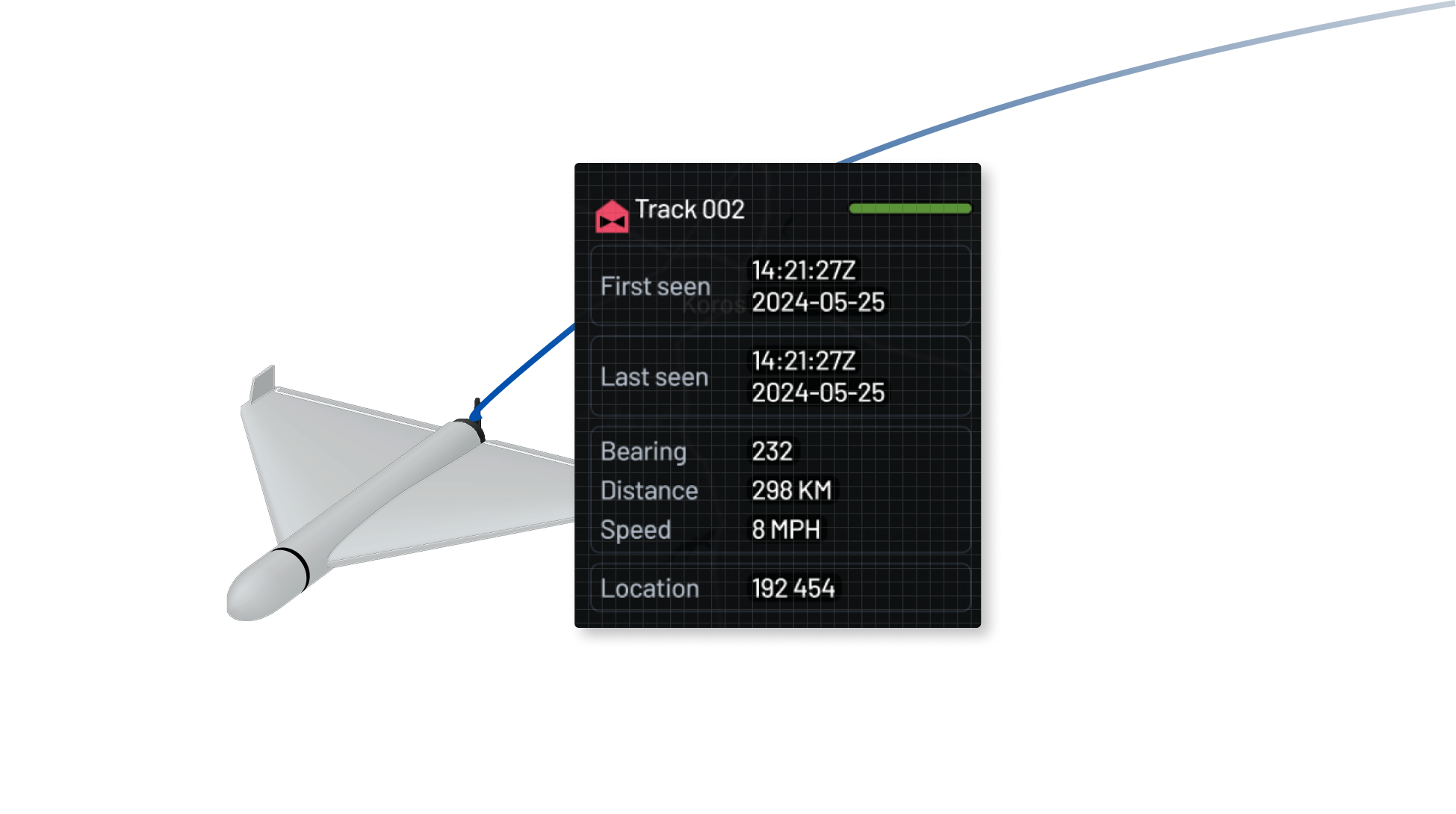
Mind Foundry Sentry
Detect Drone Intrusions with Sound
Supports early threat detection with an “acoustic tripwire” that detects, tracks, and classifies drones using their acoustic signatures.
-
Continuously detects and identifies signatures from drones in real time, filtering out environmental noises to isolate detections.
-
Ingests and processes data to precisely determine drone position and movement.
-
Detects, tracks, and classifies multiple drones simultaneously in unpredictable environments.
-
Continuous model improvement.
MIND FOUNDRY WINDWARD
Extend the Life of Ageing Infrastructure
Transforming asset inspections by combining state-of-the-art AI and Machine Learning, image correlation, and mobile-based inspection tools.
-
With just a phone in hand, engineers and inspectors can capture high-quality images, tag defects, locate issues, and log condition notes.
-
Desktop analysis to showcase every image, note, and tagged defect building a long-term view of change.
-
Visualise and measure change over time by building a digital memory that tracks assets condition from day one.
-
Use built-in templates and the data captured in the app to instantly generate professional-grade reports.
.png?width=411&height=400&name=Inspection%20App%20New%20(1).png)
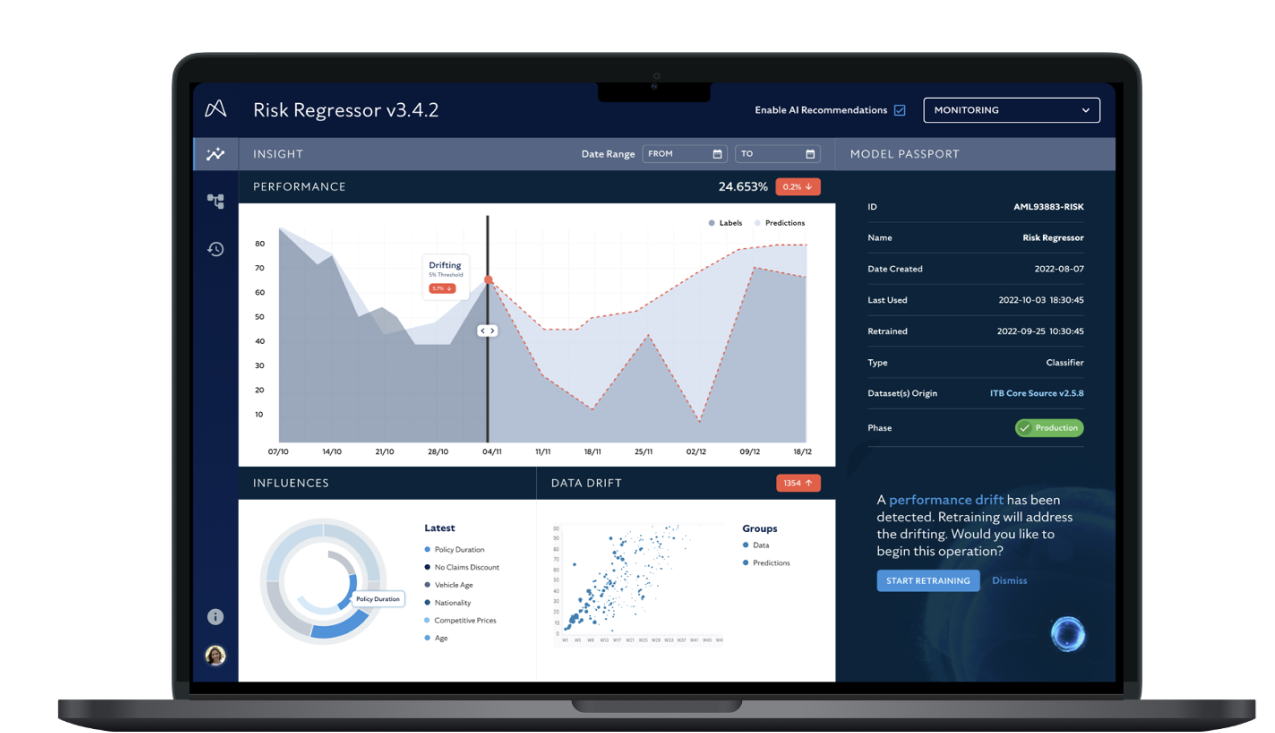
Mind Foundry Motion
Maintain and Improve Model Performance In Action
Our products and packaged service offerings for model performance and governance deliver robust assurance for the most powerful dual-use technology of our time.
-
Monitor all your models in one place.
-
Govern performance and regulatory compliance.
-
Explain your model and decision provenance.
-
Evolve and improve your AI’s capabilities over time
MIND FOUNDRY CORE
Collaborate with AI to Build Your Own Models
A platform for building and benchmarking responsible AI models.
-
Explore your data and gather insights.
-
Build models that get better over time.
-
Gain a competitive edge by developing your own IP.
-
Access technologies and tools to help across the model lifecycle.
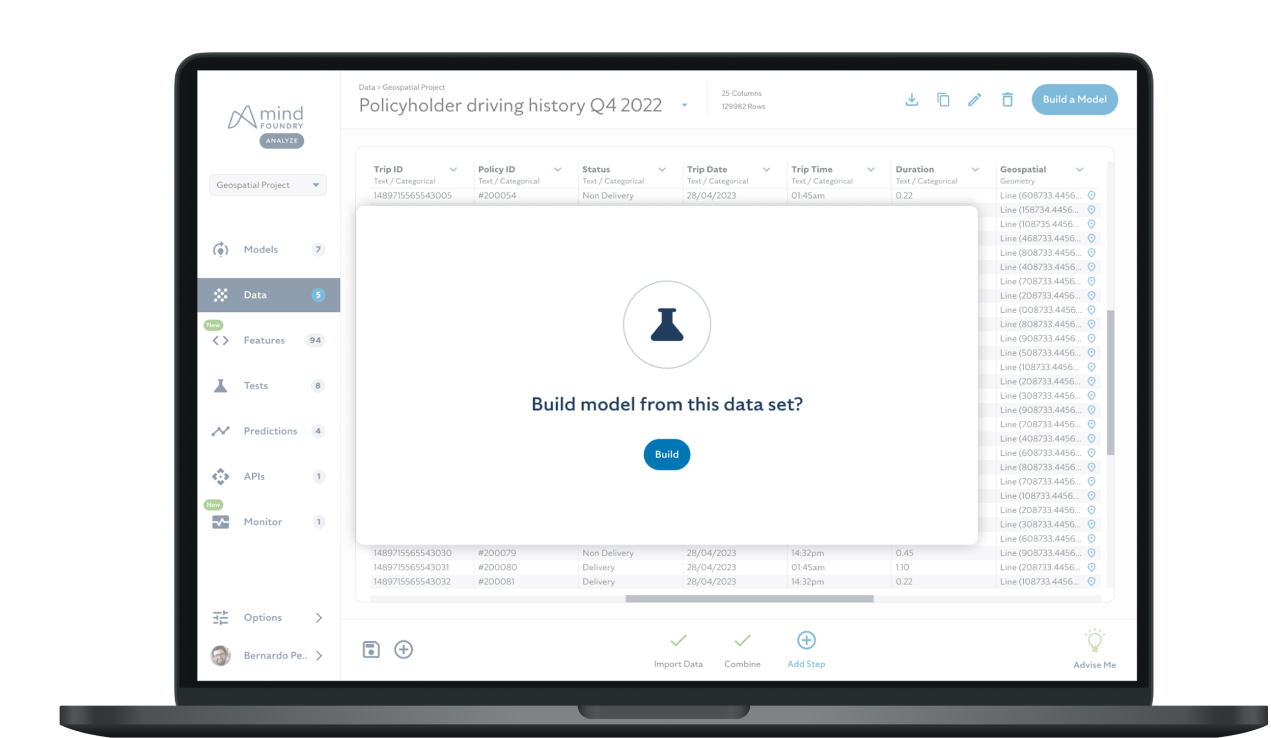
CONSULTING & BESPOKE SERVICES
Transforming your business with Machine Learning doesn't need to be complicated
The Mind Foundry Platform takes care of all the hard bits. But sometimes customisation is required: a new algorithm, an integration with a particular business process, the prioritisation of resource allocation. For all those cases, our team can help you speed up the implementation and get to results faster.
-
Dedicated team of machine learning scientists to support your needs.
-
Experience building AI solutions that really work.
-
Tried and tested processes to help you choose the right problems to solve.
-
Training, education, and road-mapping to help your digital transformation.
Education & Learning
Laying a foundation for the responsible use of AI
Creating responsible AI goes hand in hand with knowing how to use it. But as AI capability increases at machine speed, a gap is widening between current and required human capability.
With the Mind Foundry Academy and bespoke industry specific workshops, you and your team can close this gap and gain the practical knowledge to become and remain effective collaborators with AI and Machine Learning.
5 min read
AI at the Edge: Transforming Defence Operations
by Al Bowman
9 min read
The Drone Blockade: Airports Grapple with A Growing Threat
by Nick Sherman
Stay connected
News, announcements, and blogs about AI in high-stakes applications.